Bias is an error in the estimation of an association between an exposure and an outcome due to a flaw in the design or conduct of the study. Confounding on the other hand, is a real but non-causal association between the exposure and the outcome.
Although their mechanisms are different, both bias and confounding can lead to an association which is non-causal in nature between the exposure and the outcome, which results in a false conclusion when studying causality. This makes it hard to distinguish between the two.
In order to understand why bias is an error but confounding is not, let’s walk through an example where we compare confounding to selection bias.
Confounding vs Selection bias
Suppose we want to study the effect of alcohol on cancer.
The diagram below shows that:
- Smoking confounds the causal relationship between alcohol and cancer without affecting the representativeness of the study sample.
- Selection bias invalidates the causal relationship between alcohol and cancer by producing a sample that does not represent the population of interest.
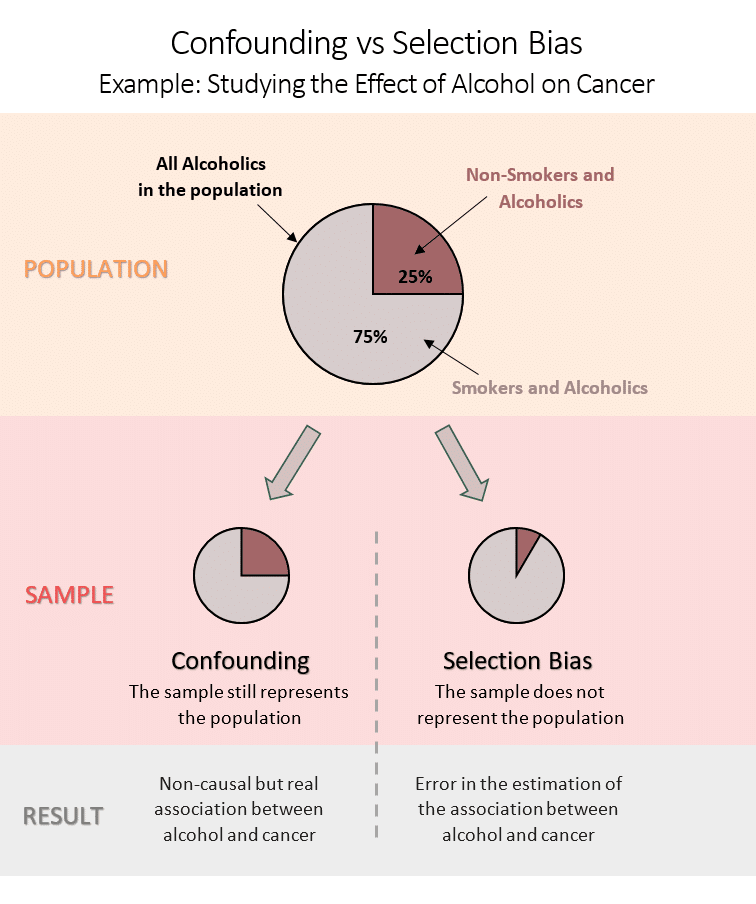
How confounding works
Because alcoholics also tend to be smokers, and because smoking is a known cause of cancer, we expect to find an association between alcohol consumption and cancer even if the 2 are not related — this is often referred to as indirect or non-causal association. In other words, the relationship between alcohol and cancer is confounded by smoking.
This association between alcohol and cancer is real however, because in the real world, alcoholics will have a higher risk of cancer (regardless of whether it is caused by alcohol itself or by confounding).
How selection bias works
Selection bias occurs when the study sample is selected in a way that causes it to be different from the population. This can happen for many reasons, but the important thing is that it leads to an error in estimating the relationship between the exposure and the outcome.
In our example above, selection bias caused smokers to be more prevalent in the study sample, and for this reason, the association between alcohol and cancer will be overestimated (since more smokers in the sample leads to a higher prevalence of cancer).
Conclusion
Similarity between confounding and bias
If we are looking to study the causal effect of alcohol on cancer, both confounding and bias will mislead us, as both will induce a non-causal association between alcohol and cancer.
In this case, the difference between confounding and bias is only of theoretical interest and does not have any practical relevance.
This is why you may find books such as: “Causal Inference: What If” and “Modern Epidemiology” that consider confounding as a type of bias.
Difference between confounding and bias
If we are working on a prevention program and we need to target high-risk groups, then it would be very important to distinguish between a biased and a confounded association.
Here’s why:
A biased association is a systematic error that leads to a false conclusion.
Systematic error means that the bias is not due to chance or to some random error.
Sampling errors due to chance tend to cancel each other out when we repeat the study multiple times. Whereas, a systematical error such as selection bias, still exists no matter how large our sample is and no matter how many times we repeat the study.
Therefore, a biased association cannot be used to do anything because it is an incorrect estimation of the relationship between the exposure and the outcome.
A confounded association however, is real in the sense that the study sample truly reflects a non-causal association that is happening at the population level and is not due to an error that the researcher has made.
For instance, if alcoholics are found to have a high risk of cancer, although that association is confounded by smoking, the association is real. This information can be used by a cancer prevention program, for example, to target the high-risk group of alcoholics in the population.
References
- Szklo M, Nieto FJ. Epidemiology: Beyond the Basics. 4th edition. Jones & Bartlett Learning; 2018.
- David Celentano, Moyses Szklo. Gordis Epidemiology. 6th edition. Elsevier; 2018.