Suppose you developed a mobile application whose aim is to help diabetic patients control their blood glucose by providing them information and practical tips on how to behave in different situations.
So you decided to design a study to figure out if this app does in fact help these patients control their blood glucose.
Here’s a simple graphical representation of the study objective:

First we will start by choosing the most appropriate design for your study. Then we will discuss the challenges you would face with each decision you make along the way.
Let’s get started!
Choosing the study design
In this section we will discuss:
- Experimental design
- Quasi-experimental design
- Which one is better for your study
1. Experimental design
In an experimental design (a.k.a. a randomized controlled trial), participants will be divided into 2 groups:
- The treatment group: The group of participants who will receive the intervention (i.e. those who will use the mobile app)
- The control group: The group of people who will not receive the intervention, but are also followed-up over time just to provide some sort of comparison/reference for the treatment group
For this design, the decision of who will be in the treatment or control group is made at random.
Because we are using randomness to decide who gets the intervention and who doesn’t and because we have a control group to compare with, the experimental design will provide the highest level of evidence of any study design.
Therefore it will always be better, when possible, to use an experimental design.
2. Quasi-experimental design
A quasi-experiment is a non-randomized study used to evaluate the effect of an intervention. So in a quasi-experiment, the decision of who gets to use the app and who doesn’t is not made at random. Instead, participants will be assigned according to their choosing or that of the researcher. Sometimes a control group will be used.
Because participants will not be assigned at random and the control group is optional, a quasi-experimental design may suffer from:
- Confounding: As the initial characteristics of the participants may provide an alternative explanation of the outcome.
- Bias: These are alternative explanations of the outcome such as: natural progression, outside events, differential selection of participants, etc.
So a quasi-experiment provides a lower level of evidence compared to a true experiment, however, it is a more practical approach when a randomized controlled trial is not feasible because of:
- Ethical considerations: If being in one group is believed to be harmful for the participant, either because the intervention is harmful (e.g. randomizing people to smoking) or has questionable efficacy or on the contrary it is believed to be so beneficial that it would be malevolent to put people in the control group (e.g. randomizing people to receiving an operation).
- Difficulty to adequately randomize subjects and locations: In some cases where interventions act on a group of people in a given location, it becomes infeasible to adequately randomize subjects (e.g. an intervention that reduces pollution in a given area).
- A small sample size: Randomized controlled trials require a large sample size to account for heterogeneity among subjects (i.e. to evenly distribute confounding variables between the treatment and control groups).
In these situations, a quasi-experimental design makes more sense than an experimental one.
3. Which design is better for your study?
A randomized controlled trial will certainly provide a higher level of evidence.
However, in your case (the mobile app study discussed above), a quasi-experiment may be a more practical approach because:
- It will be cheaper:
- since you can work with a smaller sample.
- as it does not require a control group
- You can get results faster than with a randomized controlled trial because with a quasi-experiment you may need fewer measurements and a shorter follow-up period.
So let’s suppose you decided on a quasi-experimental design without a control group.
Now you need to choose the type of quasi-experiment.
Choosing the type of quasi-experiment
Below we will discuss several types of quasi-experimental study designs without a control group (for the sake of simplicity). Note that for all of these types it would be better to add one as it would increase the level of evidence of your study.
We will start with the most simple designs that has the lowest level of evidence, moving towards more complex designs and higher levels of evidence.
1. One-group posttest-only design
In the one-group posttest-only design only 1 observation is taken after implementing the intervention. This is the weakest of the quasi-experimental designs. It is especially used when the intervention must be quickly introduced and you do not have enough time to take pre-intervention measurements.
(For more information I have a separate article on the one-group posttest-only design)
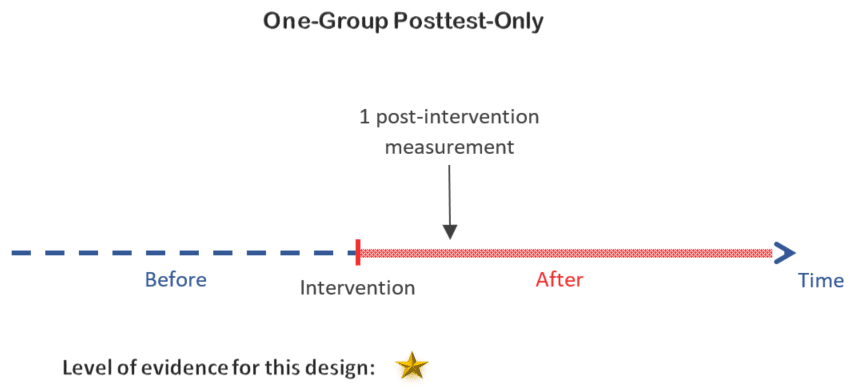
Decision:
Since taking measurements before the intervention is a possibility in your study, you can use a slightly more complex design with a better level of evidence than a one-group posttest only design.
2. One-group pretest-posttest design
The one-group pretest-posttest design is one step better than the posttest-only design. Adding a measurement before the intervention provides us with a reference value to compare our posttest results to. However, estimating the trend of the outcome with 1 pretest observation only is very weak because the outcome can be easily influenced by natural changes which cannot be taken into account in this study design.
(For more information I have a separate article on the one-group pretest-posttest design)
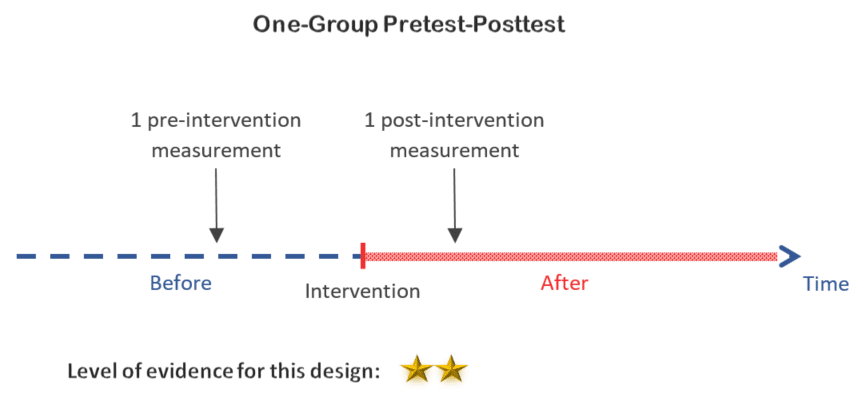
Decision:
This is a possibility, and certainly an improvement on the latter. But with 2 measurements only, it is still a weak design.
3. Removed-treatment design
In the removed treatment design, an observation is taken before the intervention, a second one after starting it, a third one before stopping the intervention and a final one after stopping it. The goal is to test if stopping the intervention has an opposite effect compared to starting it, which provides better evidence that the intervention causes a change in the outcome of interest.
Note that stopping an intervention is not always feasible especially when its effects can persist. For example if the intervention consists of providing a training on a certain subject, once learned, it would be impossible to take back information from the study participants.
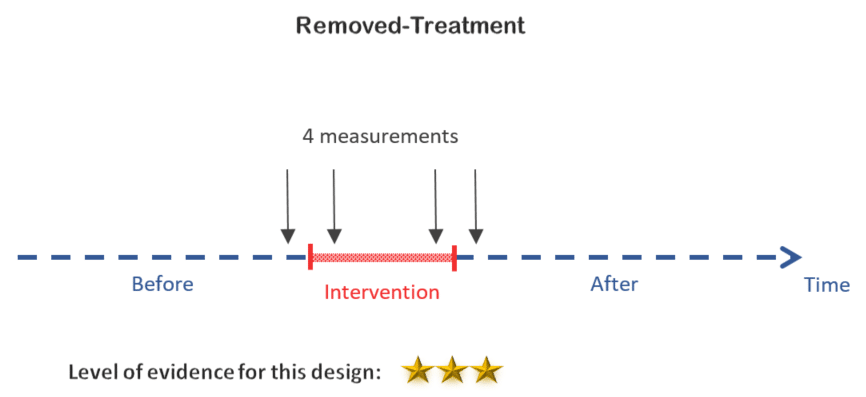
Decision:
This design is not feasible if the mobile app is providing some sort of information that can be considered as irreversible training for diabetes patients.
4. Repeated-treatment design
You can take the previous design one step further by stopping and then restarting the treatment, which provides insight on how reproducible the effects of the intervention are.
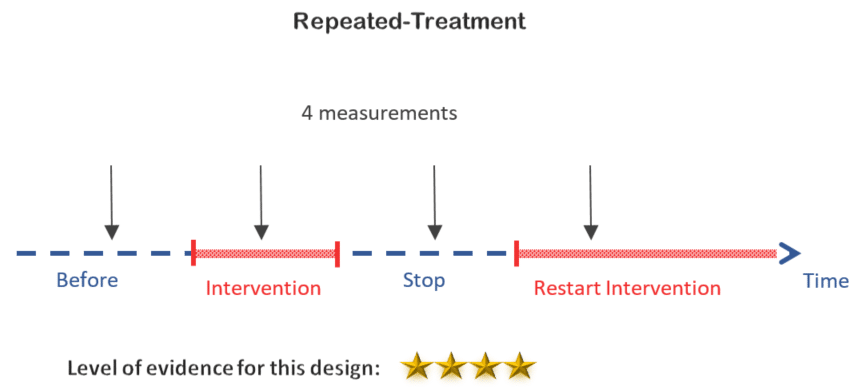
Decision:
Same as the one before, this design is not an option since the mobile app is providing some sort of information that can be considered as irreversible training for diabetics.
5. Interrupted time series design
By taking multiple measurements before and after the intervention, the interrupted time series design allows you to study the trend of the outcome thus being less vulnerable to bias from natural progression (discussed above).
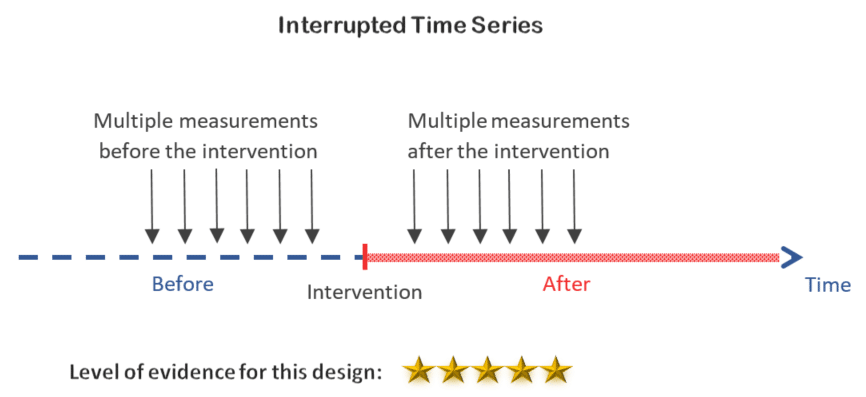
Decision:
This is certainly doable and it is the best of all the previous options.
Also if you are not limited in time and resources, you can also add a control group to get the highest level of evidence of all the quasi-experimental designs.
Confounding in a quasi-experiment
Does your study suffer from confounding? If so, how would you deal with it?
One possible source of confounding can be personality traits.
Because you did not randomly select participants to use the mobile app, it can be argued that people who would want to use a health-related mobile application may be of a certain type of personality. Also people with this personality type may be more concerned about their health than the average person, therefore more concerned about controlling their blood glucose.
Here’s a graphical representation of confounding:
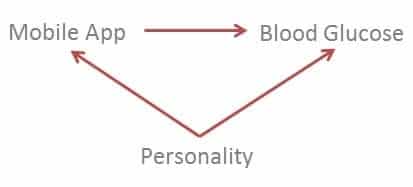
To eliminate confounding you can control for personality type for example by adding it to your linear regression model:
Blood glucose = β0 + β1 Mobile App + β2 Personality Type
For more information about confounding, I recommend these 3 articles:
- 4 Simple Ways to Identify Confounding
- 7 Different Ways to Control for Confounding
- An Example of Identifying and Adjusting for Confounding
Anyway, your study may still suffer from confounding by other unknown variables, which is why, in a quasi-experiment, it cannot be guaranteed that any correlation you find between app usage and glycemic control will certainly be causal in nature.
References
- Harris AD, McGregor JC, Perencevich EN, et al. The use and interpretation of quasi-experimental studies in medical informatics. J Am Med Inform Assoc. 2006;13(1):16–23. doi:10.1197/jamia.M1749.